[Guest Plot Post: Robert Munro is the Chief Information Officer at Energy for Opportunity and a Graduate Fellow in computational linguistics at Stanford where he specializes in methods for processing large volumes of information in less-resourced languages.]
Ushahidi platforms allow people to make order out of chaos. There is a lot of chaos. Especially during a crisis situation, large volumes of unstructured data need to be waded through to find information that is vital to the crisis affected population and to the aid organizations serving them. By the value-adding process of structuring the incoming reports (adding coordinates, categorizing, translating) the information can be quickly streamed back to those who need it most. The bottleneck is that it can take a lot of time to add structured data to unstructured reports, and people’s resources are already the most stretched during a crisis.
We are currently working with Ushahidi and CrowdFlower to address this, extending an existing collaboration in Haiti. In this new collaboration, we are working to support a team led by Faisal Chohan of BrightSpyre in Pakistan who are mapping the flood and post-flood conditions there, collecting reports from the general public and aid organizations via SMS, media monitoring and direct reports (www.pakreport.org). The potential scale of this information is extremely large, and therefore so is the potential bottleneck.
For this reason, we have built a new module for their Ushahidi deployment to ‘crowdsource the crowdsourced reports’. It is not feasible to open up the dashboard of an Ushahidi instance to too many people for reasons of scalability and security. However, we can export just the value-adding process of turning a written report into a geolocated, categorized report that is translated into one or more languages. For the deployment in Pakistan we are utilizing CrowdFlower for this process. Urdu, Pashto and English speaking volunteers from anywhere in the world can come online to the CrowdFlower task (pakreport.crowdflower.com), read one message at a time and then complete a form to add coordinates, categories and translations.
For someone managing the incoming reports with the Ushahidi deployment this processes is seamless. A report will come into the Ushahidi deployment in plain text without coordinates, translations or categories. Behind the scenes, this message is passed off to CrowdFlower for processing. Once consensus is reached in CrowdFlower, it is passed back to the Ushahidi deployment and the report is automatically updated with the structured data. Depending on the volumes of volunteers and messages, this whole process can take as little as 1 or 2 minutes.
Behind the scenes at CrowdFlower, data quality is maximized in a number of ways. Each message is passed to multiple volunteers and their responses are compared to each other for consistency. When there is great variation in responses, the task is automatically passed to more volunteers until a threshold of confidence is reached. Through this same method of cross-worker comparisons, a confidence in the overall accuracy of each individual volunteer is also calculated, which can be used as a factor in determining the overall confidence in the accuracy of the structured data for each report. For identifying coordinates, the centroid of the different coordinates is calculated, ignoring any outliers to remove the effect of individual errors. Along with the final structured report for each message, the information about response variation and confidence is also passed back to the Ushahidi deployment, allowing for a comprehensive interpretation of this processes by the core team.
Opening up this step to remote volunteers also allows for much richer interpretations of the information contained in each message. As we learned in Haiti, the crowdsourced volunteer translators were often able to identify the coordinates of addresses in emergency text messages with greater accuracy than the emergency responders. Therefore, the volunteers are also contributing their own unique knowledge to the crisis response efforts, in real-time.
It is likely that we will see more ‘crowdsourcing of croudsourced information’ in future Ushahidi deployments. CrowdMap are currently working on an API to allow the necessary interoperability and SwiftRiver are working on value-adding systems that utilize both crowdsourcing and natural language processing technologies. We are all still learning the best strategies for structuring and managing these crowdsourced integrations and work-flow processes, so we will be following the www.pakreport.org deployment closely.
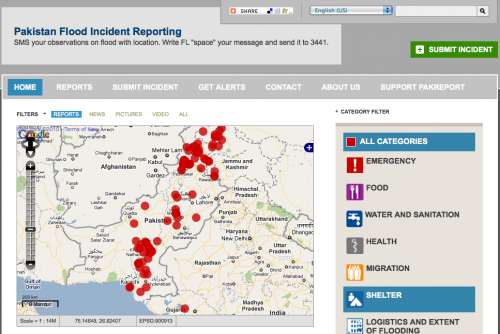
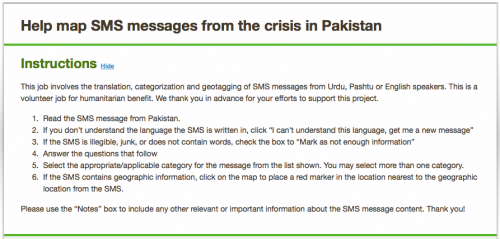